Up until now, the most commonly held definition of data literacy has been the ability to “speak” data—as if data were a language in itself that must be learned in order to present, discuss, and debate it effectively. But Generative AI (GenAI) is about to change that, making this outdated idea irrelevant.
To understand why, let’s dive into the underlying process humans have created around data. The way we collect data and the steps we go through to transform it for specific needs—whether that’s creating a dashboard or building an analytics model—has created a lot of inefficiency in organizations.
Most companies follow a process where a businessperson requests a dashboard or model, or a data analyst offers to create it. There is usually a lot of back-and-forth communication, iterating through several versions of deliverables.
As a Master Black Belt in Lean Six Sigma, I know that every business process has three versions of a process:
- What you think it is – what the process owner would like it to be.
- What it really is – how the process actually works.
- What it should be – a future state of what it can be.
The “what you think it is” version of creating a dashboard or model typically shows a detailed process with many steps, handoffs, and detailed procedures.
The “what it really is” version looks nothing like the “what you think it is” version. It looks something like this:
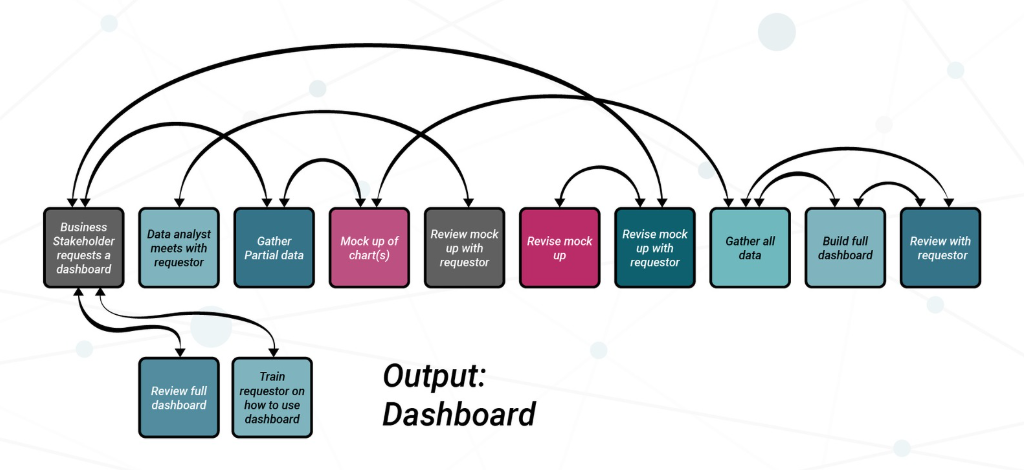
In the final steps, the data is visualized in a tool and delivered to the business stakeholder. The stakeholder views the data and hopes that it tells them something useful.
Most would agree that this process is filled with non-value-added rework, delays, and defects. Stakeholders and data experts alike are frustrated with the inefficiency. This is why I’ve always criticized the generalized “data literacy” effort—it inadvertently enables this messy, inefficient process by teaching individual role-based technical skills that promote siloed thinking and actions.
However, let’s take a look at the brighter side—what if we reimagine this process? Let’s envision the “what it should be” version, and how it sets the stage for what data literacy should truly represent today.
The New Foundation of Data Literacy: Time to Value
Instead of seeing the data process as a series of procedures, meetings, data handoffs and rework, we need to treat it as a value stream. In this new process, the goal is “time to business value”—and value is defined not by the completion of a deliverable (e.g., a dashboard). Instead, it is defined by the time it takes to deliver business value that results from the data insights.
The “Should Be” Value Stream
The output of the value stream is “actions taken” on the decisions made with insights and the quantifiable impact of those actions, such as improved revenue, cost, margin, etc. Each step is building value toward the output. In the future state, we have eliminated or reduced delays and rework because each step is focused and requires the stakeholders participation. The time-to-value is reduced by at least 50% and the likelihood of business impact is increased to 80%+ because defects are caught early in the process.
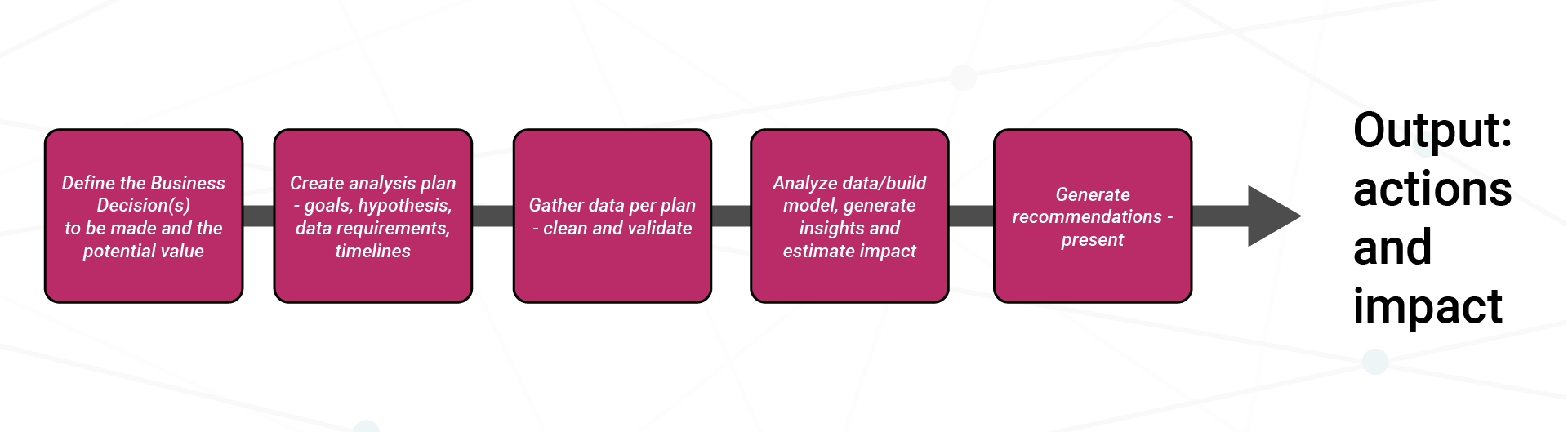
Too often, we hear requests like, “I need a chart that shows the trend of customer complaints over the last 6 months.” But, does that chart tell us what to do? Typically, no. It only leads to more questions.
Instead, we need to build a new habit of asking business questions. For example, instead of asking for data, we need to “Which complaints are driving negative CSAT scores over the past 90 days? What actions can we take to resolve complaints faster? If we solve this, what’s the potential impact on CSAT?”
This line of inquiry leads to quickly generating the right data and actionable insights. Because you can quantify the impact up front, you will know right away if the question is worth pursuing, saving valuable time and resources. Finally, the proper business question focuses on the outcome and eliminates much of the cross-functional friction over who owns what data.
How Will GenAI Change the Data Analytics Process?
Now, for the really exciting part: How will GenAI revolutionize the process? In the not-too-distant future, is this what the process will look like?
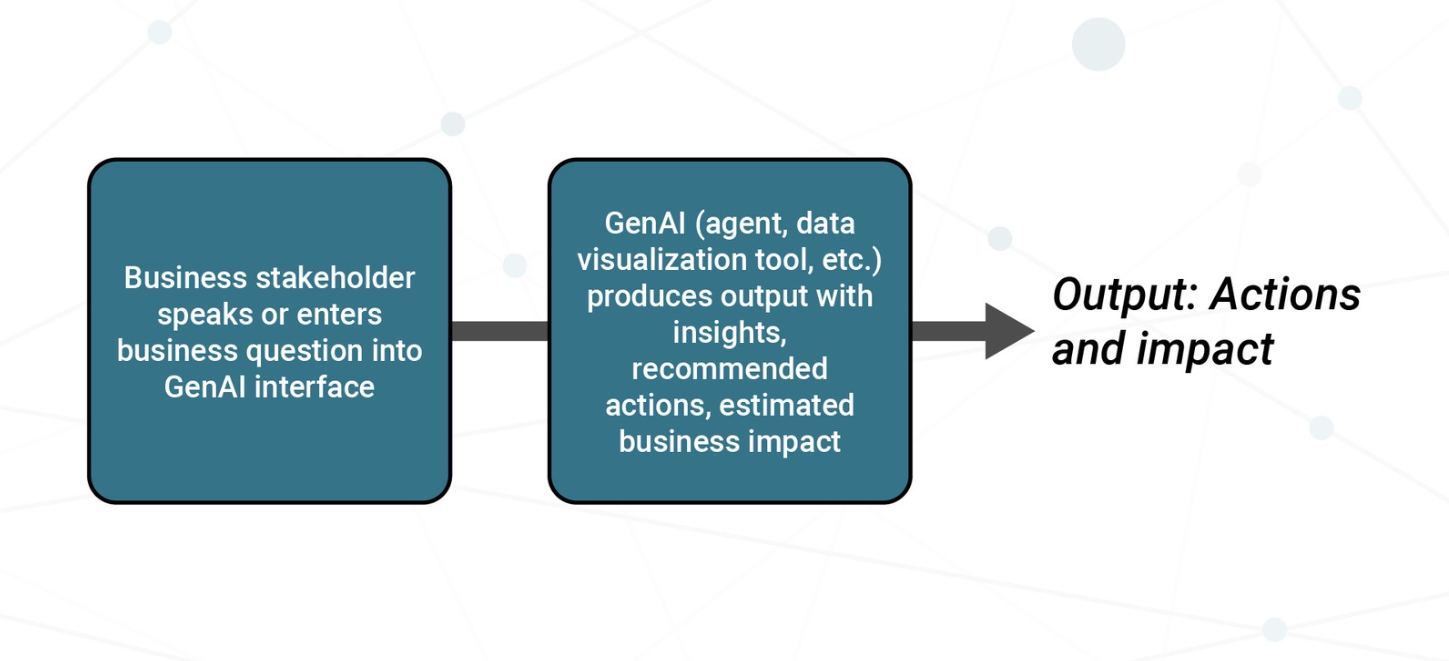
The new value stream flows much faster. It relies on critical thinking and decision-making, with a touch of computational thinking. While some of this functionality exists today, by 2026, business professionals and non-technical users will need the human skill to leverage GenAI’s capabilities to accelerate business value creation.
Data literacy will no longer be about learning to “speak data”. It will be more about “co-intelligence” in which humans frame the right business questions and making decisions. With GenAI, it will be about humans using question based prompting. Humans talk, data listens. Actionable insights, not deliverables, will be the only acceptable result.
Co-intelligence combines human decision-making with process and data/technology. You don’t have to wait for technology to develop these skills. You can start developing these habits now and apply them to your current data analytics process. You will see immediate improvements in productivity and deliver ROI more frequently. And by the time you adopt GenAI for data analytics, your people will already be prepared.
Key Steps for Transformation Leaders
As a transformation leader, there are two actions you can take right now to improve the data analytics process in your organization:
- Prioritize “time-to-value” as the key metric in your data analytics process. Shift the focus from deliverables to business outcomes.
- Create a current state, end-to-end Value Stream Mapping (VSM). Bring visibility to waste, defects, rework, and delays in the existing data analytics process. Use this VSM to define a future-state process that efficiently delivers ROI, and you’ll see quick wins right away.
Is Your Data Analytics Process Ready for the Future?
What does your current data analytics or dashboard request process look like? How long does it take to get from a business need to data insights that actually meet the decision-makers’ needs?
Most data request intake processes currently focus on maximizing the productivity of data teams or data tool experts, without measuring the true business value delivered. This results in missed opportunities and slow value creation.
The future of data analytics is all about shifting from “data literacy” as a skill to decision-making as a habit and mindset. If you want to accelerate value creation, you’ll need to start adopting this mindset today.
Authored by Susan Stocker, data literacy consultant at Aryng